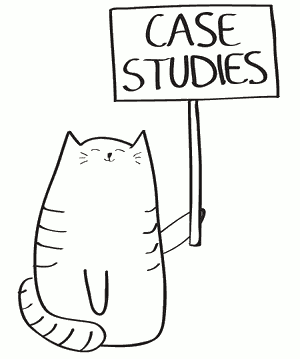
Customer Acquisition and Lead Evaluation Service
Client and requirement
A global, financial institution focused on consumer and SME credit:
- significant online and mobile presence
- provides a range of B2C and B2B digital lending products
- planning to grow its lead generator channels across Europe, North America and Asia-Pacific
- requirement to improve overall portfolio profitability and volume
- focus on sub-prime and near-prime customers.
Problem
The client faced challenges in customer acquisition and buying leads. They wanted an automated, intelligent system to answer the following questions prior to lead purchase,
- the level of lead interest?
-
how likely is the lead to:
- complete the customer journey (via website or mobile application?)
- be accepted by the decisioning and underwriting system?
- default, if a loan is granted?
- exhibit fraudulent behavior? (e.g. never pay, once the loan is granted)
- is the lead in the system already, or on a black-list?
- what is the likely retention rate and life-time-value (LTV) of the lead?
- If accepted, will the customer use company’s financial products again and how often?
- what are the predicted, risk adjusted, credit limit and terms that can be safely offered?
- is the lead prime, near-prime or a sub-prime?
- given the above, is the lead correctly priced?
- if accepted, will it be profitable?
- can you integrate easily to multiple lead generators, across countries and regions?
- can an automated system do this and still accurately filter profitable leads?
- can an automated lead evaluation system answer some or all of the above accurately in real-time?
Solution
Using the Zoral platform components listed below, Zoral provided an intelligent, automated lead evaluation system. This measured and predicted lead behavior consistently across large number of countries and regions. It provided accurate answers to the above questions using automated, real time decisioning. Initial lead volume was approximately 3000-5000 leads per day, rising to 6000 - 8000 per day.
Zoral software used
Zoral decision engine | Zoral DE |
Zoral model library | Zoral ML |
Zoral analytical data workbench | Zoral ADW |
Zoral behavioral data warehouse | Zoral BDW |
Zoral dynamic customer journey | Zoral DJ |
Implementation
Implementation was split into three phases.
Phase I
Phase 1 included the core lead evaluation functionality. For each country/region, Zoral used a range of Zoral ML models including default, fraud and limit management. (Zoral ML models are pre-tuned, highly predictive, and country/state compliant.) In addition, behavioral, credit bureau and further data sources were integrated to support AI/ML techniques.
Input
The input to Zoral DE / Zoral ML was via standard API. Data was captured from multiple lead generators. (The system was rapidly connected to more than 5 lead generators.)
Zoral DE was also connected to the client’s LMS and Black List database.
Output
Zoral DE / Zoral ML output consisted of a range of probability scores covering each of the questions described in the problem section above. These were granular and optimized for each country/region/lending product/portfolio. The output was also used to determine whether the lead provided was correctly priced before purchasing. A comprehensive range of performance statistics were also produced for each lead source. A range of real-time and periodic monitoring graphs and reports were configured using Zoral DE dashboard.
Both inputs and outputs were integrated via Zoral DE’s flexible JSON API to the client’s existing systems.
Phase I results
Phase 1 was implemented in 9 weeks. It
- lowered customer acquisition costs by 32%
- increased new customer acquisition volumes by 21%
- provided the client with the ability to grow online customer acquisition and increase geographic and regional coverage while controlling defaults
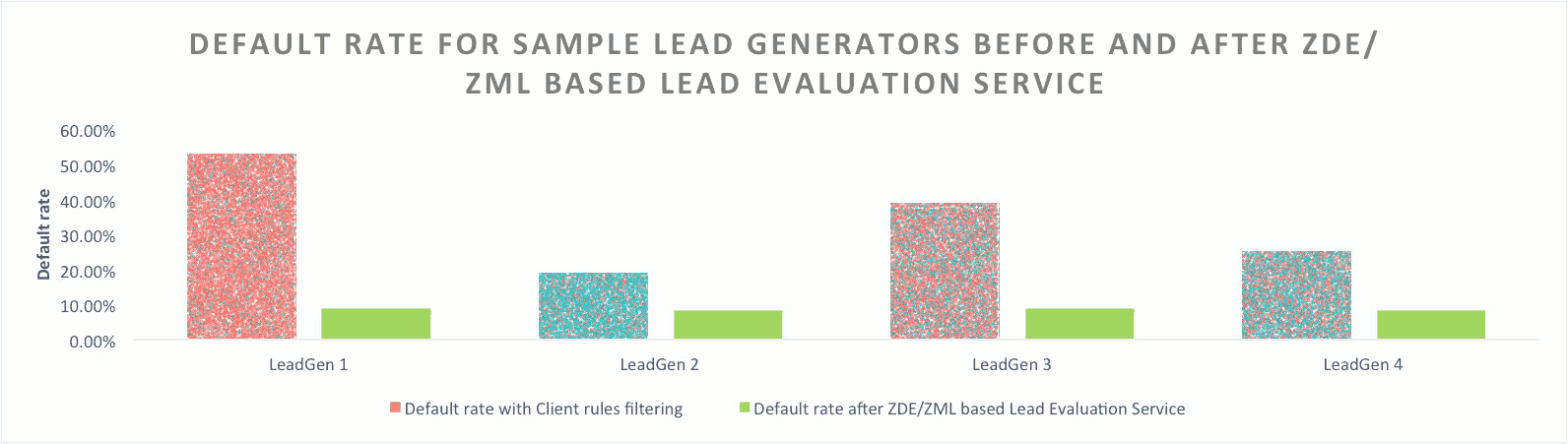
Using the Zoral platform components saved time. The system was tuned, tested, validated, calibrated and deployed within 9 weeks. It provided a fully automated, intelligent STP system. It also enabled the client to adapt quickly to subsequent geographic, market sector, and product changes.
The process of optimizing lead acquisition also exposed other issues. Certain leads with good default characteristics, (i.e. good potential customers), were not converting and were abandoning the online customer application journey. To address this issue, Zoral DE, Zoral BDW and Zoral ML were used for conversion prediction and to manage the dynamic customer journey. This was provided in Phase II
Phase II
3 months’ lead performance data was collected. This included
- behavioral
- conversion
- acceptance and
- default data.
A range of conversion models were then applied for each country, region, financial product. Lead behavioral data was captured and used as further input.
Enhanced Output
Zoral DE decision workflows were applied to predict the best product and risk adjusted offers to improve conversion. A/B tests were conducted to tailor financial products offered. Zoral DJ, Zoral BDW, Zoral DE and Zoral ML were used to dynamically personalize and optimize the customer journey.
The conversion score was also made available at lead evaluation time and was further, dynamically adjusted based on the customer’s web/mobile behavior. This enabled the system to personalize further the approach and offers for each lead.
Phase II results
- increased customer conversion rates by 27%
- increased new customer acquisition volumes by an additional 12%
- accurately measured lead generator conversion rates across countries, regions, digital financial products and lead generators.
- identified good customers who were not likely to convert and conducted A/B tests to increase conversion of these customers.
Following Phase II, the system was periodically tuned and more lead generators added. Also, some lead generators charged higher prices for good customer leads. It was hard to determine whether the lead was viable or over-priced without knowing its predicted retention and LTV. Based on client’s loan portfolio performance, Zoral ML retention and LTV models were used to provide additional, predictive lead scores. Both Retention and LTV models were further tuned after an additional 3 months’ lead data was collected for each connected lead generator.
Phase III
The objective was to measure and improve further lead retention and LTV. Using Zoral DE, Zoral deployed additional predictive Zoral ML retention and LTV models, for each country and geography implemented in Phases I and II
Input
No additional input was required.
Enhanced Output
The enhanced output consisted of a range of scores including retention score, and LTV for each country, region, product combination.
This output, in conjunction with other scores, was used by Zoral DE intelligent workflows to determine whether the lead provided was correctly priced, taking into account default, conversion, retention and LTV, before purchasing.
Phase III results
- increased new customer acquisition volume by an additional 9%
- enhanced evaluation of lead pricing prior to purchasing
- further increased overall system accuracy and acceptance rates
- improved selection and optimization of lead generators.