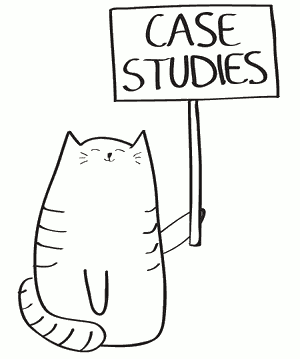
Robo-Underwriter
Client
A major, North American, consumer finance company with a substantial store network focused on the subprime and near-prime market.
The client’s products include
- short term loans
- installment loans
- lines of credit
- car financing
- savings account
- overdraft facility
- insurance
- and others.
Sub-prime lending products ranged from APR 42%-116%, with a term up to 5 years. Loan amounts ranged from $500-$5,000. Near-prime APR ranged from 26% – 38%, with a 3-5-year term, with loan amounts from $5,000 to $15,000.
The client had accumulated a substantial amount of predictive data. However, risk management, underwriting, customer lifecycle management had been built around the offline business. So operations were predominantly manual.
Operational controls were state of the art, with mature KYC validation and verification systems and procedures. Customer satisfaction was high due to a strong relationship management approach and culture.
Due to changes in the regulatory environment and other market pressures, the client, wished to,
- automate and optimize underwriting and risk management across all stores and also its online business, (robo-underwriter)
- decrease losses and improve control
- improve the performance of the existing book of business
- lower operating costs
- de-couple operating costs from portfolio size
- profitably scale online and mobile lending, expanding geographic coverage and market share
- optimize its product portfolio mix
- improve targeted marketing and customer acquisition.
The client wanted to rapidly implement intelligent automation and, at the same time, accelerate the transformation of its consumer financial products from offline to digital.
Zoral software used
Zoral decision engine | Zoral DE |
Zoral model library | Zoral ML |
Zoral loan management | Zoral LM |
Zoral analytical data workbench | Zoral ADW |
Zoral behavioral data warehouse | Zoral BDW |
Zoral MVP Implementation Approach
The implementation approach was based on Zoral‘s Minimum Viable Product (MVP) implementation methodology, followed by phased productionalization, system training and monitoring.
Zoral analyzed the offline and online loan portfolio. Zoral ML standard models were used to baseline the current business KPI’s and estimate the potential uplift possible by applying the Zoral robo-underwriter.
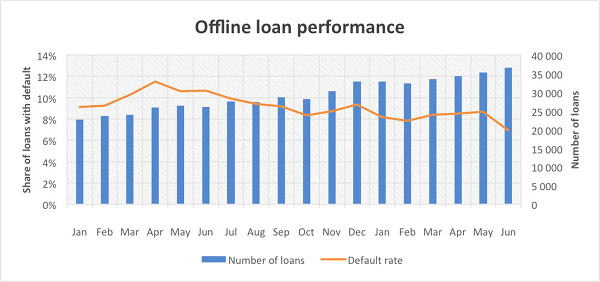
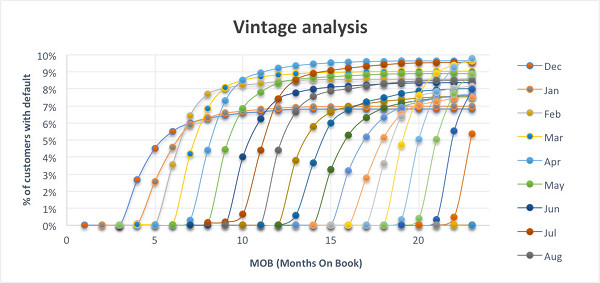
Zoral ML default models, based purely on client application data for new and returning offline customers, were applied to the portfolio. This highlighted underperforming segments in the portfolio and inefficiencies in the existing underwriting process.
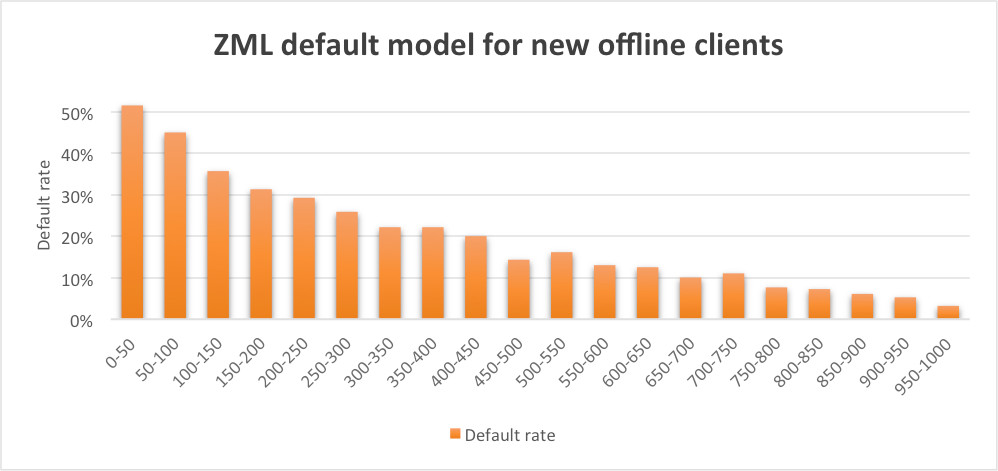
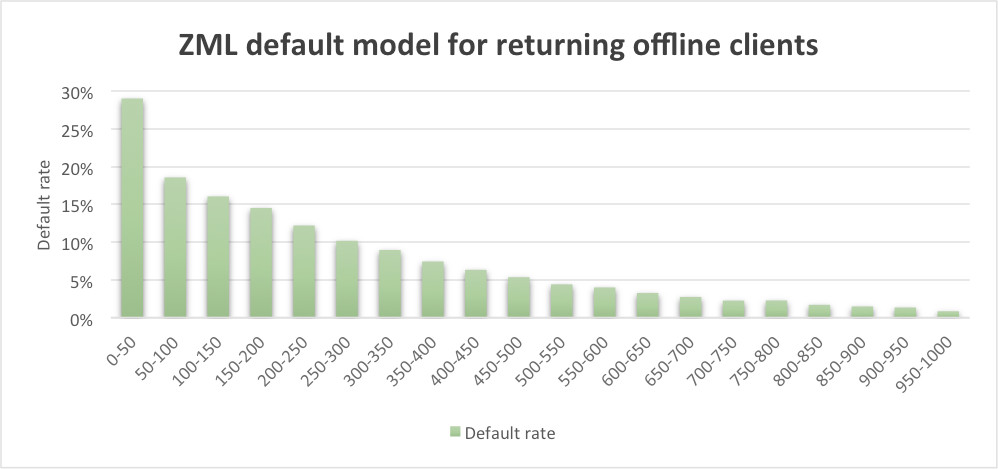
Note: each segment represents 5% of the portfolio population.
Initially, the offline portfolio was significantly larger than online. Zoral ML online default model was applied to the online portfolio, exposing underperforming segments.
After the baseline KPIs were calculated, portfolio data was enhanced. Credit bureau and bank statement data was added to the application data. This allowed the Zoral ML model capabilities to be used more fully and achieved a significant uplift in predictive quality.
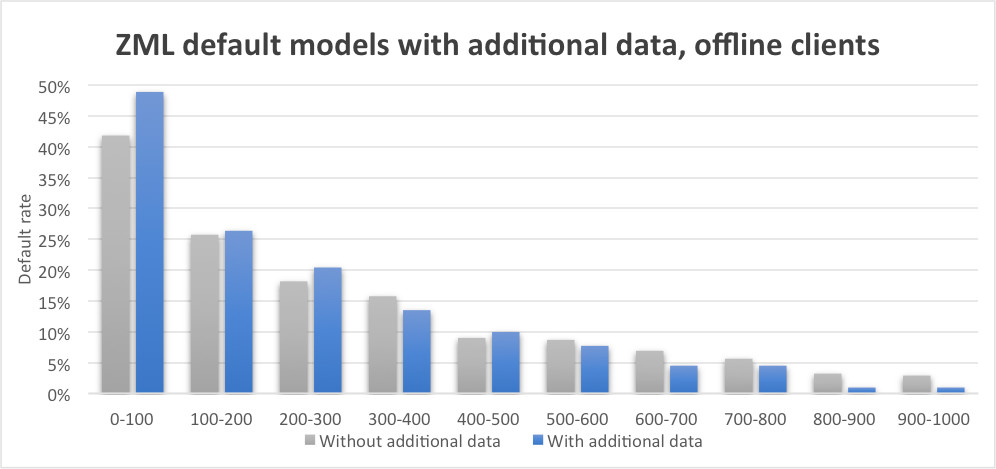
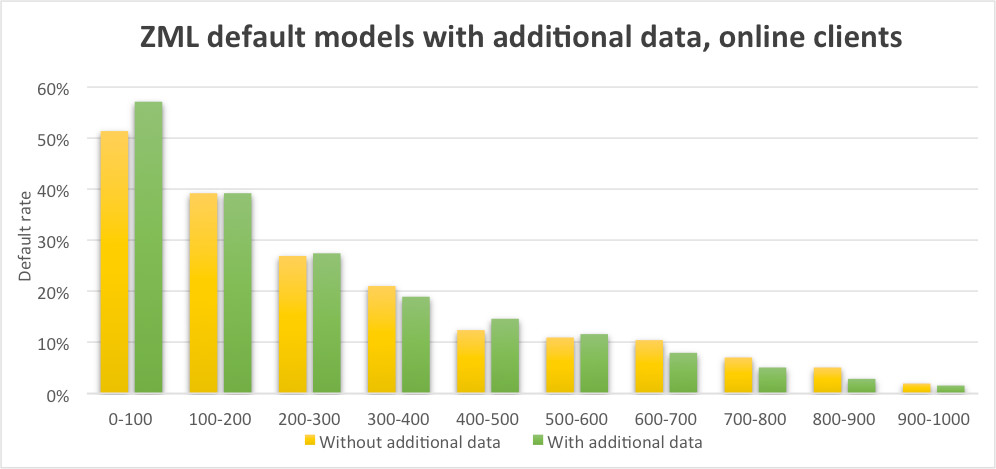
Additional fraud was discovered in both the offline and online portfolios. Zoral ML fraud models were deployed, reducing fraud. This was important as it not only reduced fraud costs, but also provided a reliable digital platform, suitable for high volume growth.
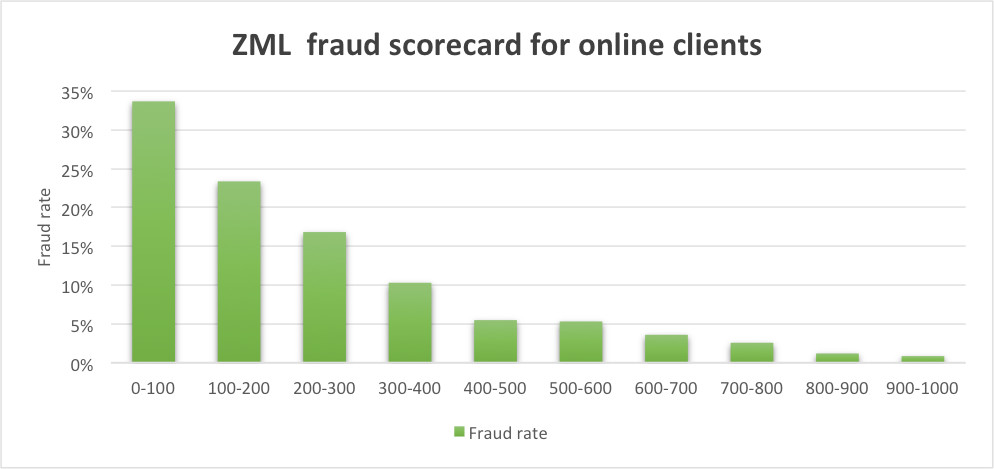
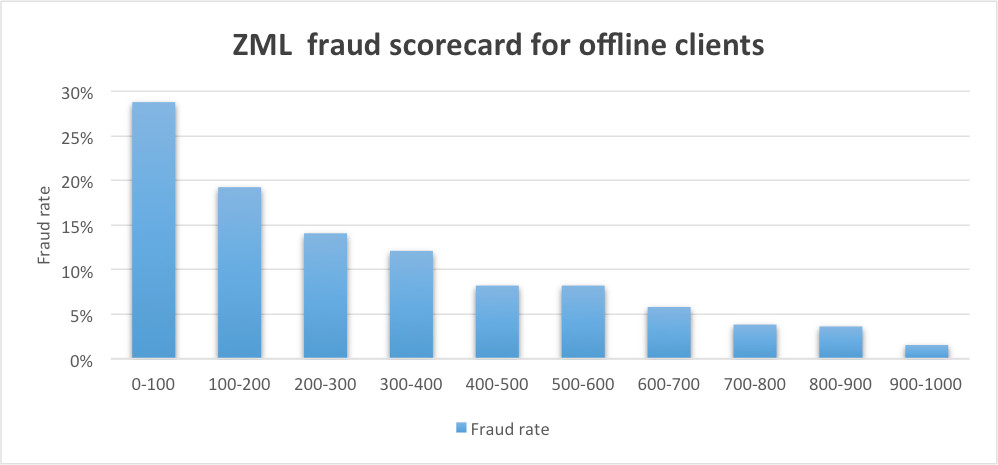
Zoral ML fraud models uncovered further fraudulent CSR activity at the stores. To address this, automated underwriting processes were introduced, substantially removing CSR’s from loan approval decisioning.
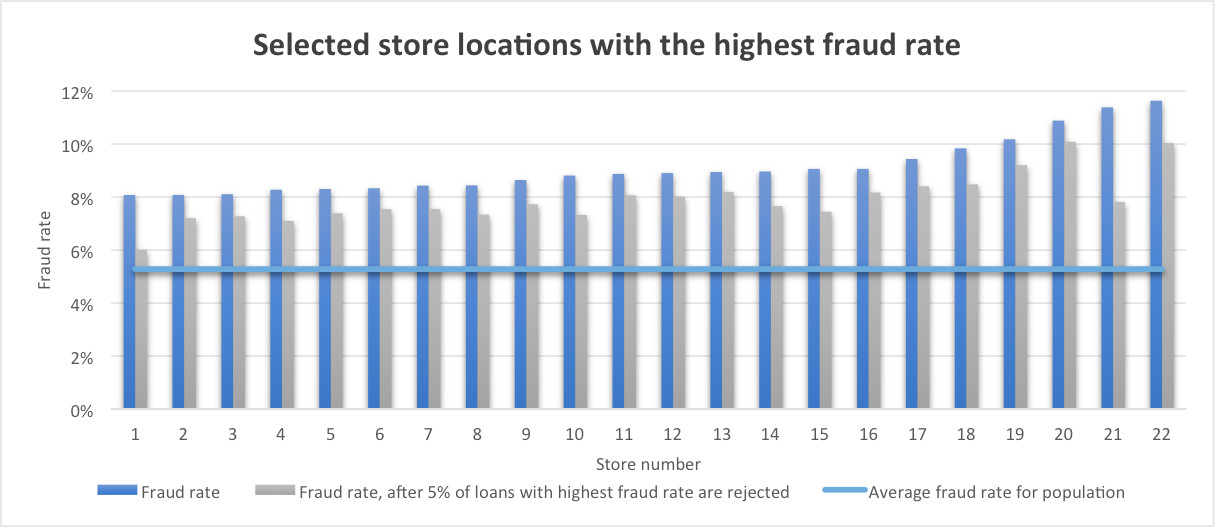
Affordability controls and models were also introduced using Zoral ML and Zoral DE. This was necessary in order to improve the quality of underwriting decisions, reduce and control frauds, and comply with the regulatory framework. This was applied to both the online and offline business.
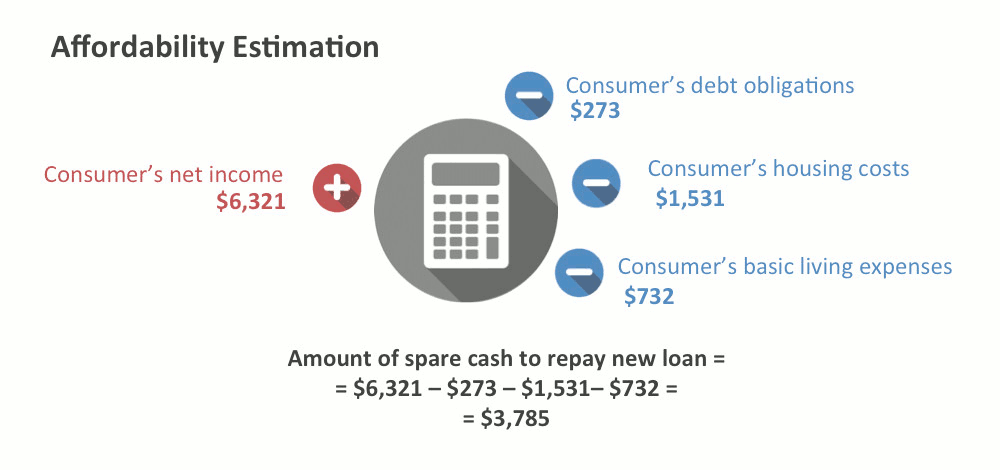
Zoral ML limit management models were also deployed for all offline and online financial products. These calculated the maximum, affordable monthly limit for each lending transaction.
Zoral DE and Zoral ML robo-underwriter
Zoral ML default, and fraud models were used as inputs to robo-underwriter to rank and segment clients based on profitability. Overall portfolio profitability was an important goal.
As well as optimizing profitability, the client wanted to keep acceptance levels as high as possible. To achieve an optimum balance, a range of Zoral ML robo-underwriter inputs were used. These included Zoral ML default, fraud, affordability control, and limit management models for offline and online product lines. Uplifts generated were significant, as can be seen from the graphs below.
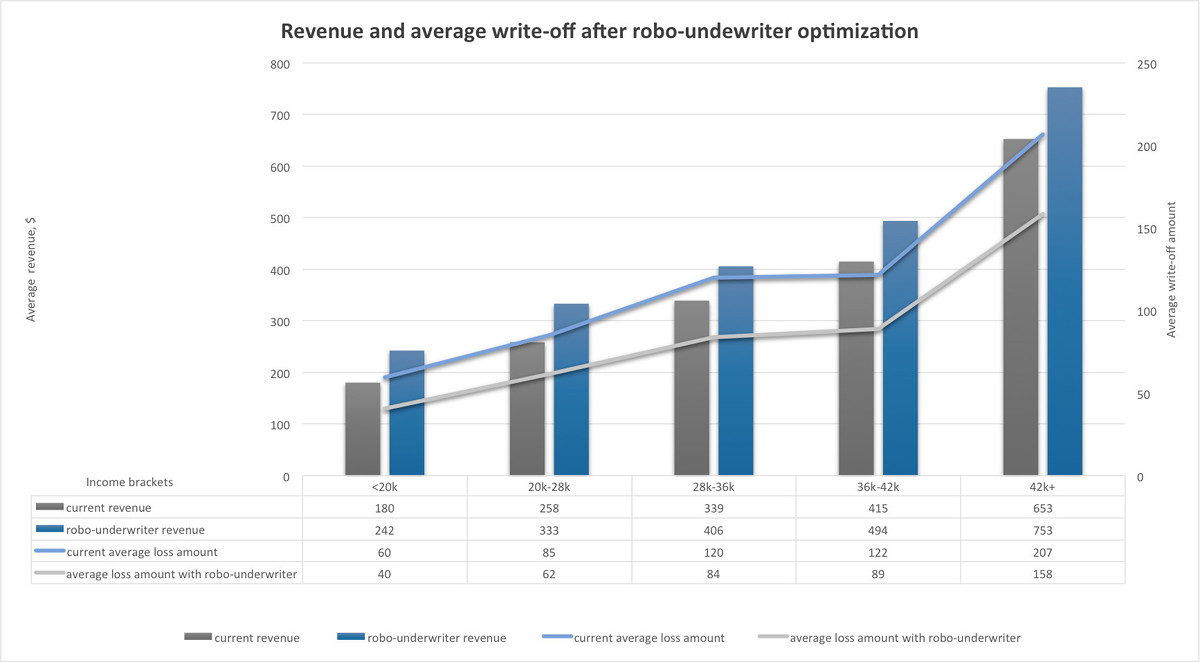
Productionalization
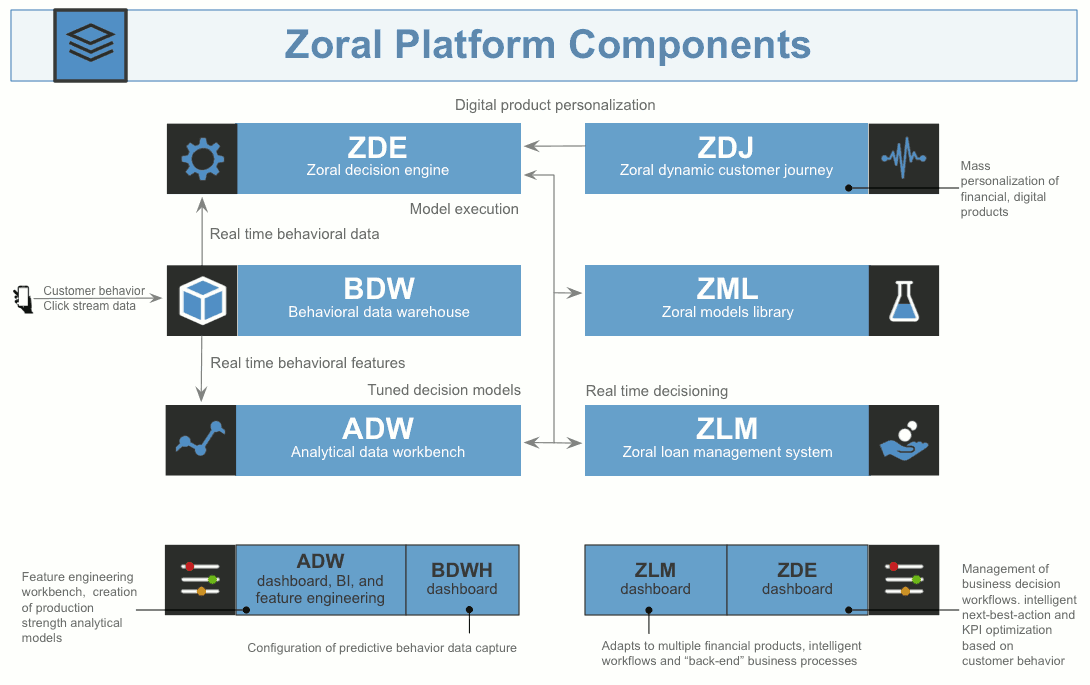
Phase I – optimize offline
- Zoral Platform was initially integrated with the client’s existing loan management system. This was done to minimize disruption to the existing business
- additional 3rd party data sources were integrated so that advanced Zoral ML models could be used
- Offline robo-underwriter was deployed across the client’s operating regions and stores
Phase II – scale online
-
Zoral Dynamic customer journey framework and intelligent business workflows were customized to fit the client’s
- brand image
- required validation
- verification
- data capture and
- business processes.
- Lead Evaluation services were added to scale online customer acquisition cost effectively and profitably
- Online robo-underwriter was deployed across the client’s operating regions
- Zoral LM was synchronized with the legacy loan management system to help achieve synergies between online and offline operations
- Behavior data capture, using Zoral BDW was implemented
Phase III – tune and optimize results
- Zoral ML conversion models were tuned and deployed as part of intelligent digital marketing workflows. This was done to optimize customer conversion across all digital channels
- Tuned Zoral ML retention models were deployed. These were used as a targeted marketing tool to improve the retention rate of profitable, existing customers
- Additional tuning was carried out once Zoral BDW was operational. The resulting behavioral data input yielded further predictive gains
- customer journey variations were A/B tested and tuned using A/I intelligent business workflows across. These helped further improve acceptance rates, profitability and customer satisfaction ratings.
During Phase III online revenue for certain products began to exceed the offline revenue.
This, in turn, allowed the client evolve other aspects of its business more rapidly, e.g. product innovation and differentiation. Operating costs were now decoupled from portfolio size growth. So the client was able to expand market share whilst containing costs and growing profitability.
The client continues to innovate, periodically introducing additional Zoral ML models and increasing automation using Zoral DE A/I intelligent workflows.
Results
The results achieved are summarized below:
- Profit increased by 28%
- Portfolio write-off decrease by 19%
- quarterly volume growth increased by 12%
- online STP increase from 50% to 87%
- customer acquisition costs reduced by 32%
- customer retention rate increased by 9%
- online conversion rate increased by 31%
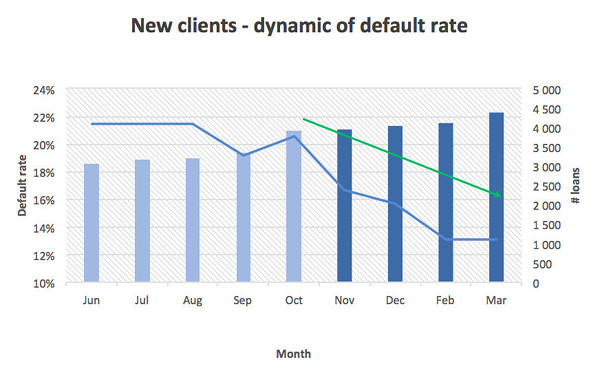
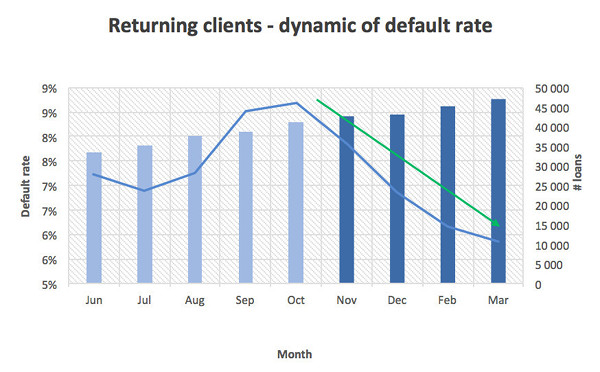
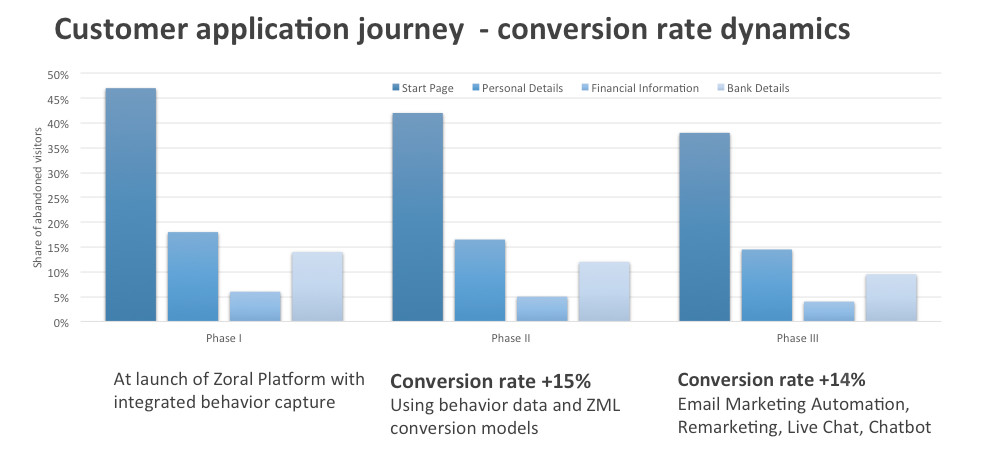