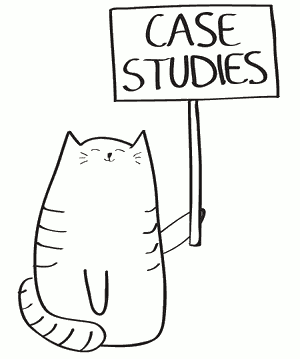
Zoral DE/ML Robo-Underwriting
Intelligent automation of SME loan underwriting
Automation has the potential to bring four main benefits:
- the ability to increase substantially loan volumes without increasing staff
- reduce operating costs
- improve on overall portfolio profitability
- achieve consistent underwriting quality across all SME loans.
The following case study explains how this was achieved for a major, North American, SME lender by using Zoral DE and Zoral ML.
The client and the problem
The client is a leading North American SME finance company. Their objective was to grow substantially their SME loan portfolio and market share. This presented a challenge. Their current underwriting processes were sophisticated and effective. However, they were traditional, manually intensive and difficult to scale.
Also, as SME lending and underwriting required substantial analytical knowledge and experience, underwriting performance was not consistent across the underwriter team.
Manual underwriting and quality control processes were supported by a set of loosely integrated systems. These, in turn, used manual information capture and semi-manual work flows.
Lack of underwriting automation caused an increase in time to process loans as the SME lender business tried to scale.
The effect of growing volumes, without systematic underwriting automation, started to impact and reduce decision quality and consistency.
The client had rapidly expanded the underwriting team to try and cope. However, this led to deteriorating portfolio results. It became clear that the underwriting process was a bottleneck and needed to be automated.
The requirement

To solve the above problems the client needed to,
- automate its underwriting using a mix of human and machine intelligence
- reduce operational costs whilst maintaining growth
- automate and improve risk adjusted pricing and portfolio management rigor
- improve data quality, make better use of existing data and adding new data sources
- improve the quality of underwriting decisions and acceptance rates
- reduce and better control loan loss provisions
- add more digital products to its range.
The solution chosen was Zoral DE / Zoral ML robo-underwriting platform. This was used to implement intelligent underwriting automation. In turn, this also helped to accelerate moving off-line products to digital.
Zoral software used
Zoral decision engine | Zoral DE |
Zoral model library | Zoral ML |
Zoral analytical data workbench | Zoral ADW |
Implementation Approach
The implementation approach was based on using standard Zoral DE and Zoral ML products and Zoral‘s Minimum Viable Product (MVP) implementation methodology. Implementation was completed in 15 weeks, including phased integration to client systems and 3rd party data sources, deployment to production, handover and systems training.
Zoral Decision Engine was configured to integrate with:
- SME application and documentation data captured
- client’s internal CRM and Deal Rooms
- core banking/financial and LMS systems
- Yodlee/Decision Logic
- multiple credit bureau (D&B, Experian, etc.)
- external, 3rd party, comprehensive SME/company time-series databases
- government, and 3rd party SME verification services
- Zoral ML SME Website data extraction,
- Zoral Social Data Extraction Service
Zoral ML intelligent, robo-underwriter workflow was tuned and optimized for maximum effect. This was done using automatically enriched, predictive SME data.
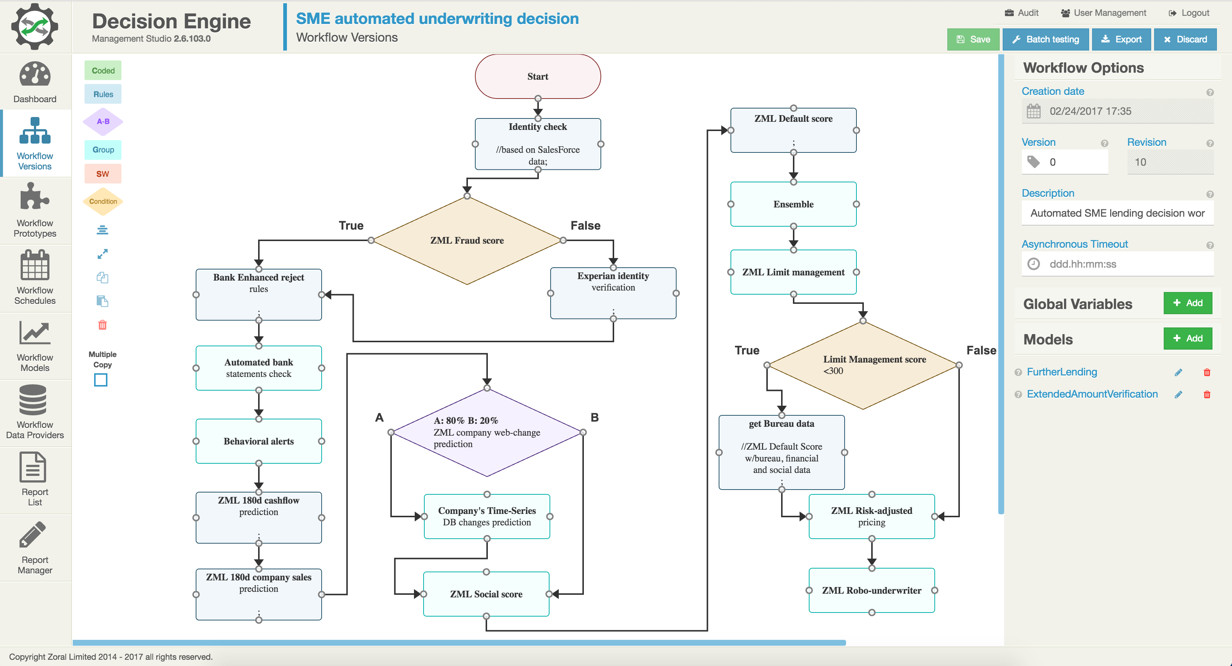
A range of Zoral ML standard models were used.
The use of Social Data, Zoral ML Social Score, and Zoral DE intelligent workflows as part of the SME Finance company system architecture is shown below.
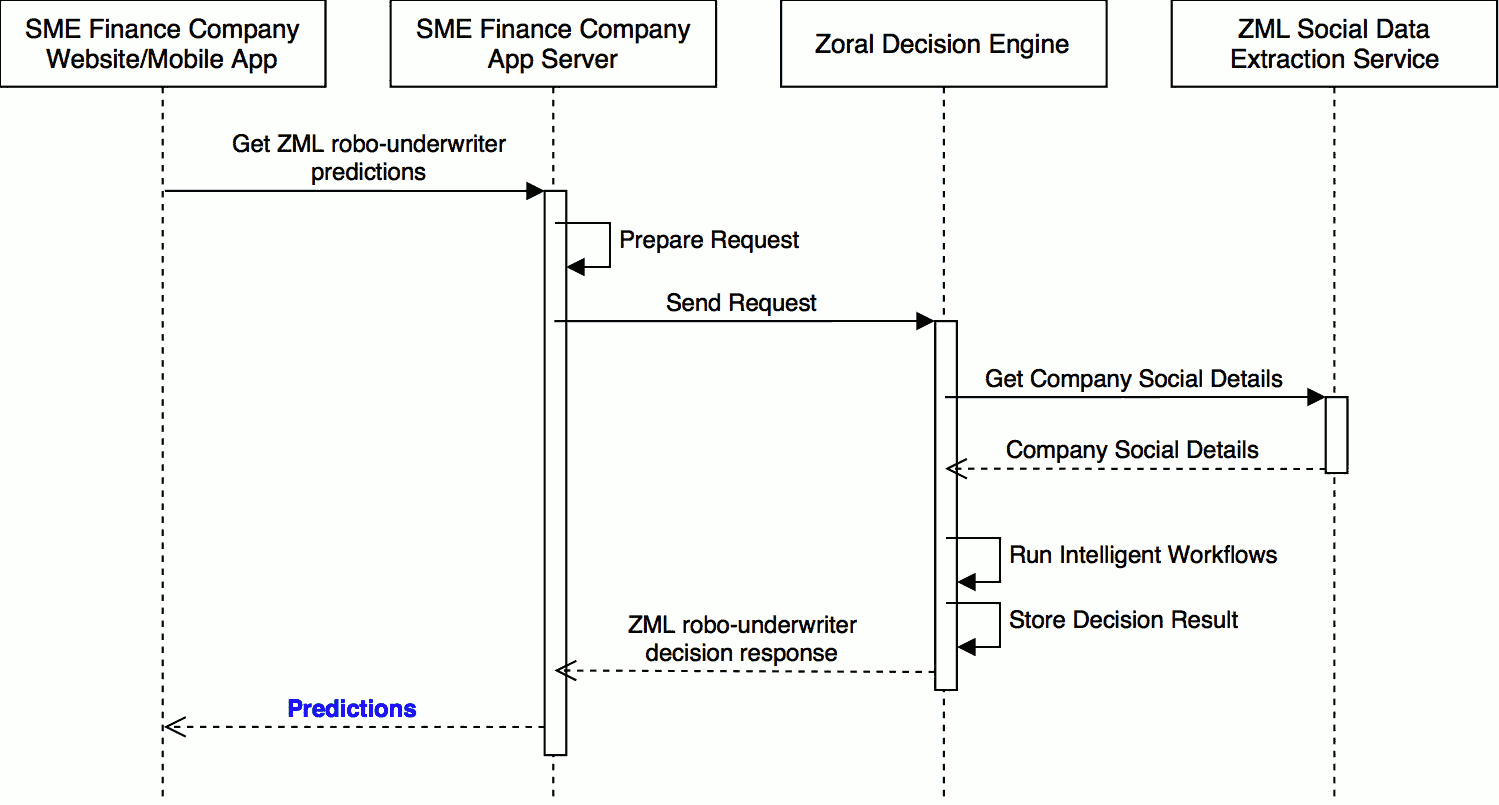
Default and Write-off prediction
Zoral ML default model was used to predict write-offs automatically.
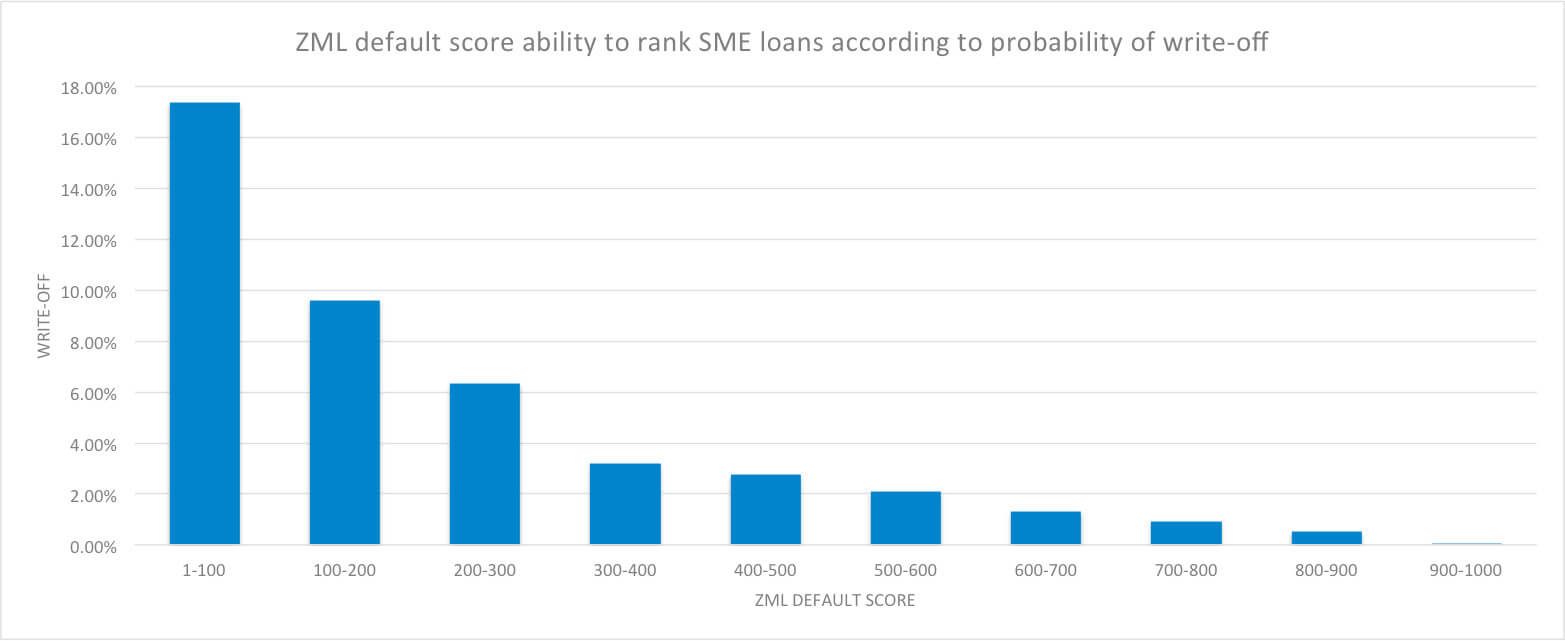
Note: each segment represents 10% of the SME lending portfolio population.
Initially, only raw, existing data was used to benchmark the internal underwriting score against Zoral ML default score. Additional data sources were added subsequently. This resulted in immediate improvements in overall accuracy, as can be seen below.
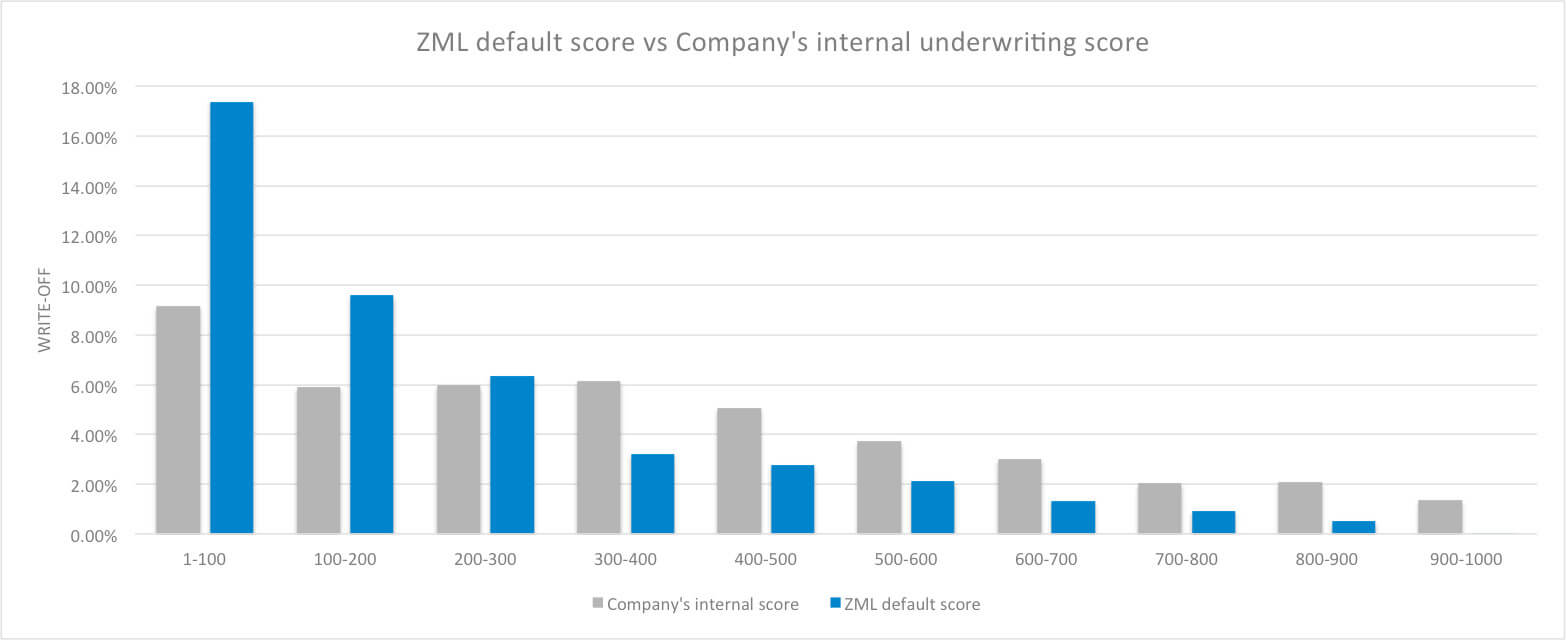
Note: Zoral Platform shows higher predictive power as compared to client’s internal default prediction model. This is largely due to three factors: (1) ADW allowed a far wider range or predictive data to be discovered (e.g. 100’s of data points as input vs. client’s existing, human tuned models, which used less then 30 input variables) (2) Zoral DE used, multiple ensemble, non-linear models vs. the client’s traditional, linear model techniques (3) Zoral ML ADW contained many hundred’s of man years R&D and global experience and embedded knowledge.
The client’s data was enriched automatically using predictive social data and Zoral Social Score.
This improved write-off prediction as shown in the graph below.
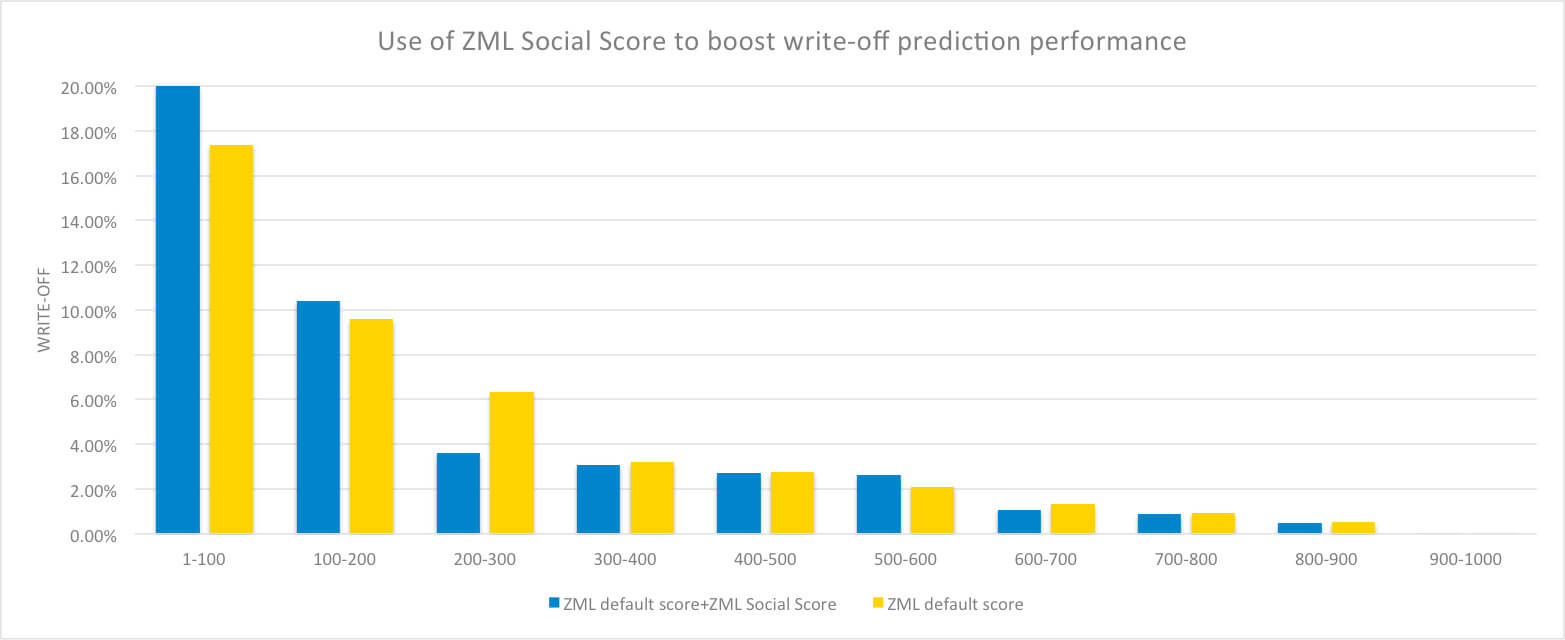
Next, underwriting department performance was analyzed, using Zoral DE and Zoral ML default models,
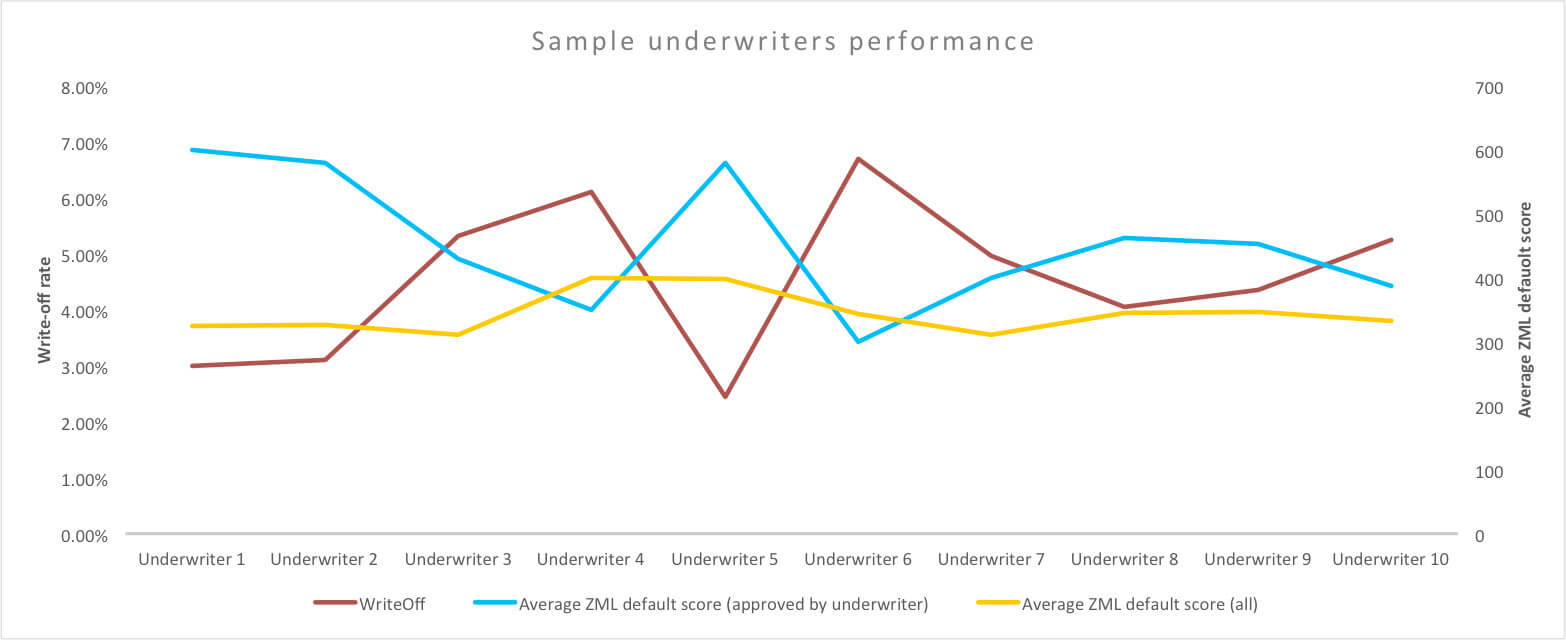
Note: A representative sample of the clients SME loan underwriting department performance over latest one year period is shown. The blue line shows the average default rate of approved SME loans by each underwriter in the sample. Underwriters operated using the same underwriting policies, and approval procedures, however, the average default rates and corresponding write-off rates (shown in red) varied widely.
As can be seen on the graph above, the performance/metrics of the best underwriters was good, but overall performance was not consistent across the team, as shown below.
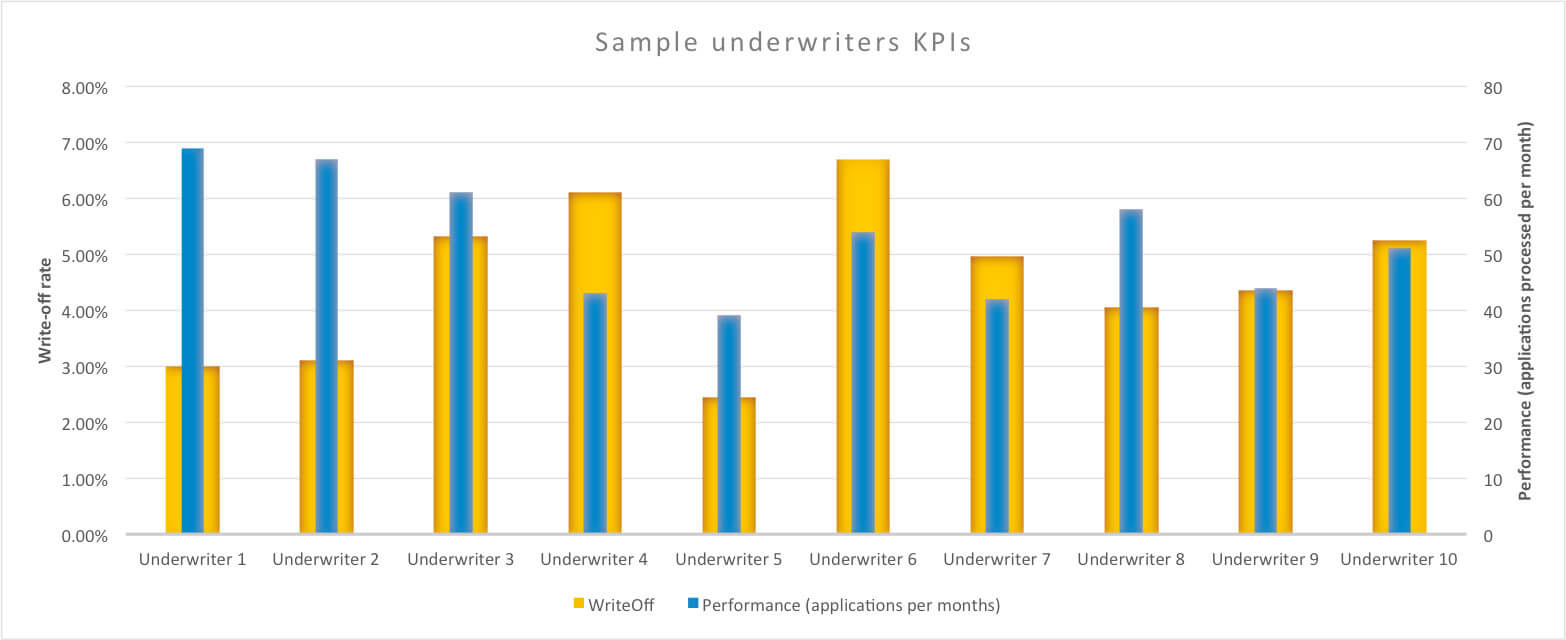
Note: Most productive underwriter #6, (who was given mostly $20,000 - $50,000 SME loans to review and underwrite), approved, on the average, 67 loans per month. The least productive and very experienced underwriter #5, on similar types of loans, approved on the average 24 loans per month but with a much lower write-off.
There were other variations in SME loan write-off performance by underwriter. These were caused by the underwriters using their internally calculated score as a starting point and then adding their own expert judgment across the five C’s of credit, (character, capacity, capital, collateral, and conditions). Using this process, they arrived at an adjusted classification of SME loan risk.
A comparison was run, using Zoral DE and Zoral ML, of underwriter collective classification of SME lending risk. This was compared to an automated Zoral DE / Zoral ML default score based classification of the same risks for a period of a year. This revealed further performance deterioration as shown below.
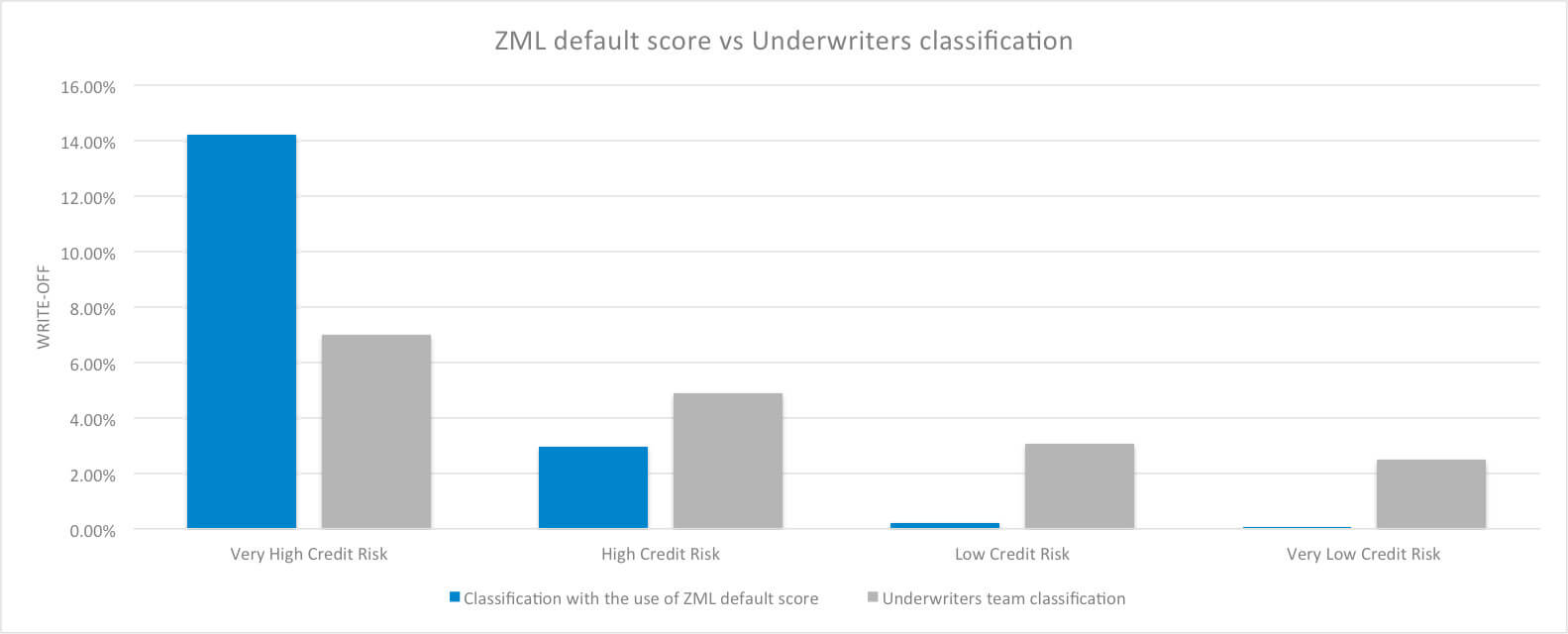
Despite variations in underwriter performance shown, the overall portfolio write-off and profitability performance was acceptable for a “steady state” business. However, this did not support the growth being experienced and anticipated. Put simply, the client’s underwriting processes and systems could not scale rapidly or safely. A larger proportion of underwriting decisions needed to be automated and better use made of a wider range of predictive customer and 3rd party data.
Next steps
A larger range of Zoral ML models and automated SME verifications were introduced. Their results were used as inputs into Zoral DE robo-underwriter to support automated and semi-automated underwriting procedures. Zoral ML robo-underwriter outputs were then integrated via Zoral DE to the client’s existing systems to provide automated underwriting decisioning. These covered the entire system lifecycle, from lead arrival through to underwriting approval and loan fulfillment. The results were,
- best underwriters made even better decisions
- “weaker” underwriter performance improved
- the underwriter team was able to scale to much larger transaction volumes
- portfolio metrics improved
- less money and time were spent on leads that either did not close, or performed poorly
- client underwriting scalability goals were achieved
- 95% of loans under $50,000 were automatically underwritten, using Zoral DE and Zoral ML robo-underwriter
- optimal risk adjusted pricing and terms was applied
- the improvement in automation freed the underwriting team from a large amount of work and allowed them to focus manual efforts on larger/”exceptional” loan opportunities and new products.
The client was able to transition from traditional to artificial intelligence and machine learning underwriting for the majority of its portfolio.
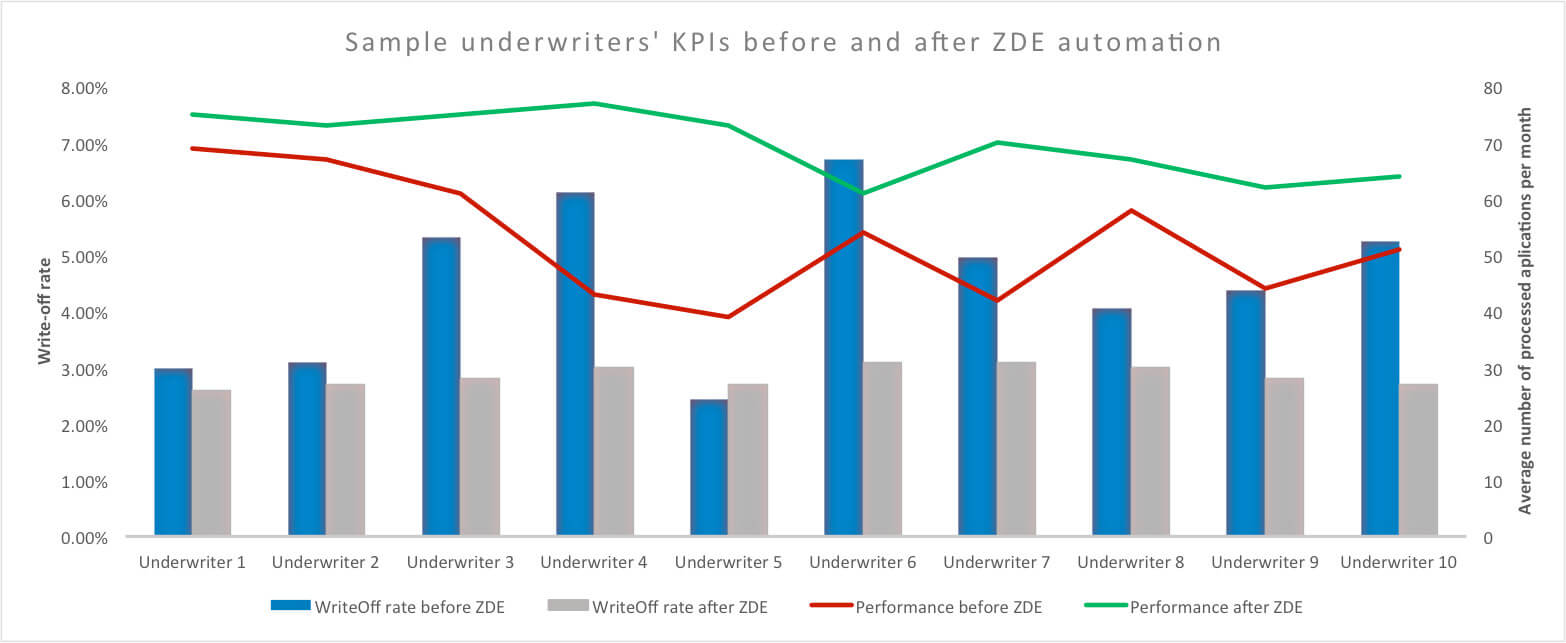
Robo-underwriter automation achieved with the use of Zoral DE and Zoral ML models. The SME loan portfolio write-off rate decreased. Write-off rate variations, leveraging the Zoral ML robo-underwriter, shown in grey, were significantly reduced, resulting in the “weaker” underwriter’s performance rising with increased monthly loan volumes.
Results
The results achieved are summarized below:
- Profit increased by 27%
- Portfolio write-off decrease by 68%
- Yearly volume growth increased by 123%
- Manual underwriting decreased by 70%
- Acceptance rate increased by 16%
- Variation of underwriter performance decreased
- Transitioned 95% of portfolio underwriting decisions from traditional to artificial intelligence and machine learning underwriting for SME loans under $50,000